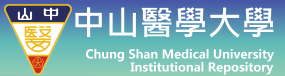 |
English
|
正體中文
|
简体中文
|
Items with full text/Total items : 17938/22957 (78%)
Visitors : 7398037
Online Users : 276
|
|
|
Loading...
|
Please use this identifier to cite or link to this item:
https://ir.csmu.edu.tw:8080/ir/handle/310902500/24009
|
Title: | AEP-DLA: Adverse Event Prediction in Hospitalized Adult Patients Using Deep Learning Algorithms |
Authors: | Wu, CL;Wu, MJ;Chen, LC;Lo, YC;Huang, CC;Yu, HH;Pardeshi, MS;Lo, WT;Sheu, RK |
Keywords: | Adverse event (AE);early deterioration indication;early warning scores;electronic medical record;risk stratification |
Date: | 2021 |
Issue Date: | 2022-08-05T09:46:22Z (UTC)
|
Publisher: | IEEE-INST ELECTRICAL ELECTRONICS ENGINEERS INC |
ISSN: | 2169-3536 |
Abstract: | Early prediction of clinical deterioration such as adverse events (AEs), improves patient safety. National Early Warning Score (NEWS) is widely used to predict AEs based on the aggregation of 6 physiological parameters. We took the same parameters as the features for AE prediction using deep learning algorithms (AEP-DLA) among hospitalized adult patients. The aim of this study is to get better performance than traditional naive mathematical calculations by introducing novel vital sign data preprocessing schemes. We retrospectively collected the data from our electronic medical record data warehouse (2007 similar to 2017). AE rate of all 99,861 admissions was 6.2%. The dataset was divided into training and testing datasets from 2007-2015 and 2016-2017 respectively. In real-life clinical care, physiological parameters were not recorded every hour and missed frequently, for example, Glasgow Coma Scale (GCS). The expert domain suggested that missed GCS was rated as 15. We took two strategies (stack series records and align by hour) in the data preprocessing and tripling the values of negative samples for class balancing (CB). We used the last 28 hours' serial data to predict AEs 3 hours later with Random Forest, XGBoost, Convolutional Neural Network (CNN) and Recurrent Neural Network (RNN). It is shown that CNN with CB and align by hour got the best results comparing to the other methods. The precision, recall and area under curve were 0.841, 0.928 and 0.995 respectively. The performance of the model is also better than those proposed in the published literatures. |
URI: | http://dx.doi.org/10.1109/ACCESS.2021.3070618 https://www.webofscience.com/wos/woscc/full-record/WOS:000641015900001 https://ir.csmu.edu.tw:8080/handle/310902500/24009 |
Relation: | IEEE ACCESS ,2021,v9 , P55673-55689 |
Appears in Collections: | [中山醫學大學研究成果] 期刊論文
|
Files in This Item:
File |
Description |
Size | Format | |
index.html | | 0Kb | HTML | 208 | View/Open |
|
All items in CSMUIR are protected by copyright, with all rights reserved.
|