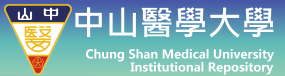 |
English
|
正體中文
|
简体中文
|
Items with full text/Total items : 17938/22957 (78%)
Visitors : 7396351
Online Users : 233
|
|
|
Loading...
|
Please use this identifier to cite or link to this item:
https://ir.csmu.edu.tw:8080/ir/handle/310902500/23609
|
Title: | End-to-end deep learning for recognition of ploidy status using time-lapse videos |
Authors: | Lee, CI;Su, YR;Chen, CH;Chang, TA;Kuo, EES;Zheng, WL;Hsieh, WT;Huang, CC;Lee, MS;Liu, M |
Keywords: | Time-lapse;Deep learning;Preimplantation genetic testing for aneuploidy (PGT-A);Ploidy status |
Date: | 2021 |
Issue Date: | 2022-08-05T09:40:03Z (UTC)
|
Publisher: | SPRINGER/PLENUM PUBLISHERS |
ISSN: | 1058-0468 |
Abstract: | Purpose Our retrospective study is to investigate an end-to-end deep learning model in identifying ploidy status through raw time-lapse video. Methods By randomly dividing the dataset of time-lapse videos with known outcome of preimplantation genetic testing for aneuploidy (PGT-A), a deep learning model on raw videos was trained by the 80% dataset, and used to test the remaining 20%, by feeding time-lapse videos as input and the PGT-A prediction as output. The performance was measured by an average area under the curve (AUC) of the receiver operating characteristic curve. Result(s) With 690 sets of time-lapse video image, combined with PGT-A results, our deep learning model has achieved an AUC of 0.74 from the test dataset (138 videos), in discriminating between aneuploid embryos (group 1) and others (group 2, including euploid and mosaic embryos). Conclusion Our model demonstrated a proof of concept and potential in recognizing the ploidy status of tested embryos. A larger scale and further optimization on the exclusion criteria would be included in our future investigation, as well as prospective approach. |
URI: | http://dx.doi.org/10.1007/s10815-021-02228-8 https://www.webofscience.com/wos/woscc/full-record/WOS:000652922400001 https://ir.csmu.edu.tw:8080/handle/310902500/23609 |
Relation: | JOURNAL OF ASSISTED REPRODUCTION AND GENETICS ,2021,v38,issue 7, P1655-1663 |
Appears in Collections: | [中山醫學大學研究成果] 期刊論文
|
Files in This Item:
File |
Description |
Size | Format | |
index.html | | 0Kb | HTML | 152 | View/Open |
|
All items in CSMUIR are protected by copyright, with all rights reserved.
|