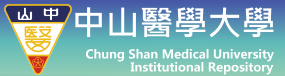 |
English
|
正體中文
|
简体中文
|
Items with full text/Total items : 17933/22952 (78%)
Visitors : 7359488
Online Users : 470
|
|
|
Loading...
|
Please use this identifier to cite or link to this item:
https://ir.csmu.edu.tw:8080/ir/handle/310902500/23454
|
Title: | The Clinical Influence after Implementation of Convolutional Neural Network-Based Software for Diabetic Retinopathy Detection in the Primary Care Setting |
Authors: | Li, YH;Sheu, WHH;Chou, CC;Lin, CH;Cheng, YS;Wang, CY;Wu, CL;Lee, IT |
Keywords: | area under the curve;diabetes;deep learning;image;retinopathy |
Date: | 2021 |
Issue Date: | 2022-08-05T09:37:33Z (UTC)
|
Publisher: | MDPI |
Abstract: | Deep learning-based software is developed to assist physicians in terms of diagnosis; however, its clinical application is still under investigation. We integrated deep-learning-based software for diabetic retinopathy (DR) grading into the clinical workflow of an endocrinology department where endocrinologists grade for retinal images and evaluated the influence of its implementation. A total of 1432 images from 716 patients and 1400 images from 700 patients were collected before and after implementation, respectively. Using the grading by ophthalmologists as the reference standard, the sensitivity, specificity, and area under the receiver operating characteristic curve (AUC) to detect referable DR (RDR) were 0.91 (0.87-0.96), 0.90 (0.87-0.92), and 0.90 (0.87-0.93) at the image level; and 0.91 (0.81-0.97), 0.84 (0.80-0.87), and 0.87 (0.83-0.91) at the patient level. The monthly RDR rate dropped from 55.1% to 43.0% after implementation. The monthly percentage of finishing grading within the allotted time increased from 66.8% to 77.6%. There was a wide range of agreement values between the software and endocrinologists after implementation (kappa values of 0.17-0.65). In conclusion, we observed the clinical influence of deep-learning-based software on graders without the retinal subspecialty. However, the validation using images from local datasets is recommended before clinical implementation. |
URI: | http://dx.doi.org/10.3390/life11030200 https://www.webofscience.com/wos/woscc/full-record/WOS:000633825300001 https://ir.csmu.edu.tw:8080/handle/310902500/23454 |
Relation: | LIFE-BASEL ,2021,v11,issue 3 |
Appears in Collections: | [中山醫學大學研究成果] 期刊論文
|
Files in This Item:
File |
Description |
Size | Format | |
index.html | | 0Kb | HTML | 183 | View/Open |
|
All items in CSMUIR are protected by copyright, with all rights reserved.
|